The birth of ChatGPT fired the first shot of the modern AI arms race. The large language models represented by GPT-4, ChatGTP, Bard, etc. have attracted widespread attention from all walks of life around the world. Combined with the underlying technical logic of ChatGPT, there are four general directions for ChatGPT industrialization in the short to medium term: namely, intelligent customer service, AIGC applications of text modality, code development-related work, and image generation. Among them, the most suitable project for direct landing is the intelligent customer service work.
Intelligent customer service built on large model technology is fundamentally changing the traditional human-computer interaction process. Large models automatically generate dialogue processes to make operating intelligent customer service more efficient, improving the rate of complex winding problems, the degree of human-computer interaction perception, and the efficiency of operational content such as intent understanding, process construction, and knowledge generation.
If we look at the product penetration rate alone, intelligent customer service has been slowly spreading in e-commerce, finance and other fields in the past seven to eight years. The two core changes brought about by the big model are a significant decrease in the cost of developing intelligent customer service products and an improvement in user experience.
Around 2016, it took a small team of seven or eight people several months to complete a prototype of intelligent customer service. With a large model, it can now be done by one engineer in two to three days. Although it takes longer to productize a prototype product, it is an order of magnitude decrease compared to the previous investment in labor and time costs.
In addition, although the intelligent customer service products in the past were called "intelligent", they gave users the impression of being "dull" when answering questions, and basically the answers were pre-written templates, and the questions they could answer were limited. Now the big language model can give personalized answers according to the user's questions and the corresponding standard answers, and it is not easy to tell whether it is an artificial customer or a machine customer service in the user experience, which is an obvious improvement.
With the breakthrough of the new generation of intelligent technology, especially the large model that can provide powerful natural language generation capability for intelligent customer service, it is bound to push intelligent customer service to a new situation that is more intelligent, efficient and personalized.
However, it must be noted that although the application prospect of large language models in the field of intelligent customer service looks very optimistic, the application scenario of large language models is still immature at this stage: the main manifestation is that the generated results are not stable and the reliability is not high enough. In terms of practical application scenarios, if a mature and market-proven product is rashly introduced into the model, it may bring huge changes to the product and even affect customer experience and waste a lot of human effort.
Model unveiling in the field of intelligent customer service
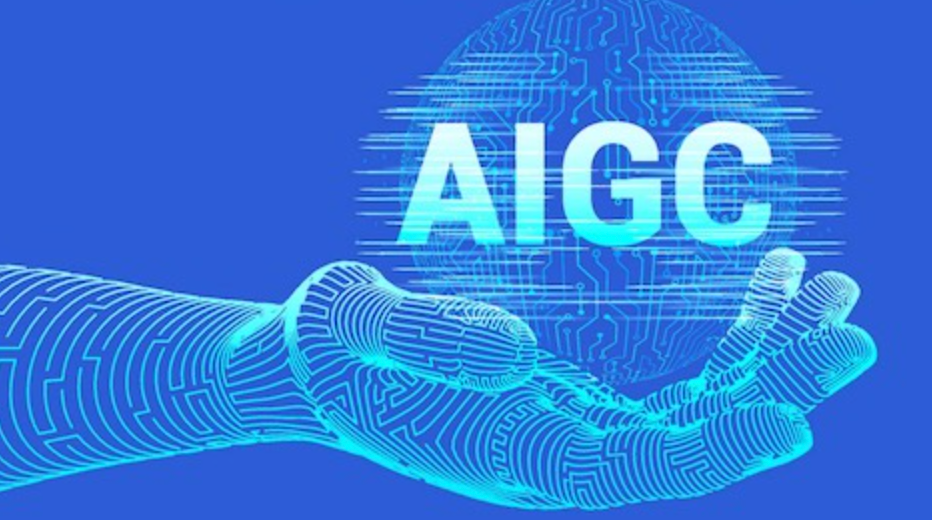
At present, the advantages of AIGC intelligent customer service are very obvious, and with the continuous upgrading and improvement of AIGC intelligent customer service technology, the prospect of its application in the fields of metallurgy, e-commerce and logistics is also very optimistic.
However, before the big model sets off a "new revolution" revolution, we still need to pave a good road for it and cannot be too hasty. For example, in the field of intelligent customer service products, conversations are usually limited to specific business areas and task-driven needs. But customers don't want visitors to come in just looking for a super-smart, understanding, writing and drawing chatbot. Conversational content must converge within the business context of the enterprise, serving customer service and marketing scenarios, solving and handling problems, and not wasting valuable resources. Introducing big models like ChatGPT directly into the customer service domain without control will inevitably lead to disappointing results.
Building a big model that can be applied to business is not an easy task, and usually requires several steps, including big model selection, data collection and cleaning, model training, model testing and evaluation, model fine-tuning, and application deployment. Specific links:
- Select open source generic big models
- Collect domain data, use the domain data to continue training the open source big model (academically called knowledge injection), and get the domain big model
- Organize the instruction set of domain tasks (can be generated semi-automatically), and use the instruction data to fine-tune the domain big model
- Screening high-quality customer service multi-round conversation data of real scenarios, and continuing to fine-tune the domain big model (to enhance the conversation capability of the domain big model)
- Collect some security-related questions (e.g. involving politics, sensitive topics, discrimination, etc.), manually give answers that meet security requirements, and continue to fine-tune the big model (aligning to human values, norms)
- Model deployment, docking to intelligent customer service system for application
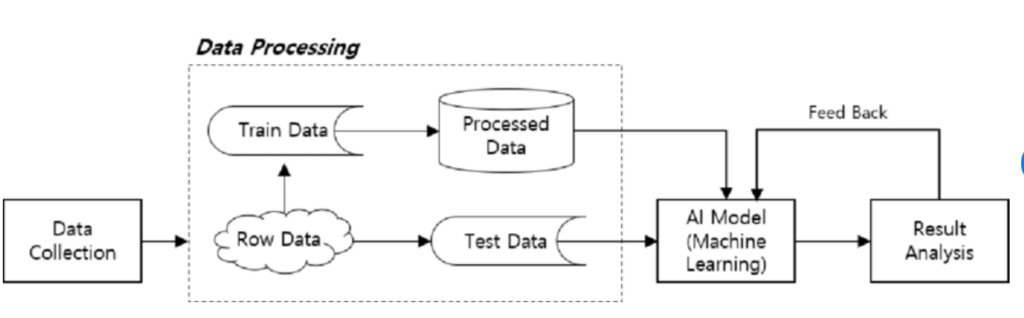
Data Preparation
The training data of the dialogue model should be of sufficient quality and diversity to improve the generalization ability of the model. Conversation data can be obtained through various ways such as crawlers, Q&A communities, and conversation records. After obtaining the data, data cleaning and pre-processing are needed to make the data in standardized format and high quality.
Model Training
After preparing the training data, it is time to start training the large model. During the training process, appropriate hyperparameters, such as learning rate, batch size, and number of training rounds, need to be selected to achieve the best results. In addition, distributed training techniques can be used during the training process to speed up the training process.
Model Optimization
After the model training is completed, the model needs to be evaluated and optimized to improve the generalization ability and effectiveness of the model. Common optimization methods include adjusting hyperparameters, adding training data, using regularization techniques, pruning models, etc. In addition, techniques such as model distillation can also be used to transfer the knowledge from large models to small models to improve the effectiveness of small models.
Conversation generation
After the models are trained and optimized, dialogue generation can be performed. Dialogue generation can be achieved in two ways: one is to use a single model for dialogue generation, and the other is to use multiple models for dialogue generation. When using a single model for dialogue generation, the dialogue model needs to have the ability to remember, i.e., the model can generate more reasonable and coherent dialogue responses by contextual information. When using multiple models for dialogue generation, the diversity of models can be used to generate richer and more diverse dialogue texts.
In an intelligent customer service product, there is no place where content productivity is more important than the knowledge base. Generally, intelligent customer service products have such knowledge bases: internal knowledge bases, machine knowledge bases, and external knowledge bases.
For internal knowledge bases, they are generally defined as knowledge bases that need to be used for real-time positioning queries. As the business of an enterprise changes frequently, the adjustment of the knowledge base needs to be in place in a timely manner. The traditional processes of uploading, editing, and organizing are very work-intensive. The introduction of a large model can help employees categorize and create the categories and details of the knowledge base in a highly efficient and intelligent way. At the same time, it can also increase the use of external data sources and reduce the synchronization of knowledge bases. Users can also give feedback on knowledge points and help the knowledge base adjust weights automatically when they are used in practice.
The knowledge base of machine mythology is the core of text and voice machines that can answer visitors' questions. The effective content of the knowledge base is crucial to the performance of the machine. For sorting out unknown questions, intelligent customer service users need to put in a large amount of work. Automatically organizing and correlating unknown questions with user feedback can save a lot of work for knowledge base maintainers. At the same time, through the combination of multiple machine myths, they can take over different scenarios of customers in a single session, and the large model of exclusive machine myths can also play a role in specific occasions and gradually replace some question-and-answer machine myths with rigid patterns in the past.
The external knowledge base needs to be integrated into intelligent customer service products, and the organized knowledge content can be transformed into output products, which can be more easily created into knowledge files, pictures, or even audio and video. Based on ChatGPT's multimodal AIGC capability, a personalized knowledge space can be created quickly.
Generally speaking, we use the large model's own creation capability, based on vector database and trusted content review technology, to provide high-quality content to supplement intelligent customer service.
Although the process and chain of building large models in the field of intelligent customer service are basically clear, there are still various challenges in the actual implementation process.
These challenges mainly come from the data level, as well as the level of interfacing with the actual customer system scenarios, the product itself is rather easy. For example, the customer data of the new system to be put on is itself raw data and needs to be cleaned up with high quality and structured data to have a better result, but this step is very difficult.
The second one is that when actually going deeper into the business, there will be various information scattered in various systems within the customer, and only when these information are opened up can there be a good effect and experience. These are the challenges that need to be tackled in the future.
How to make intelligent customer service speak "human language"?
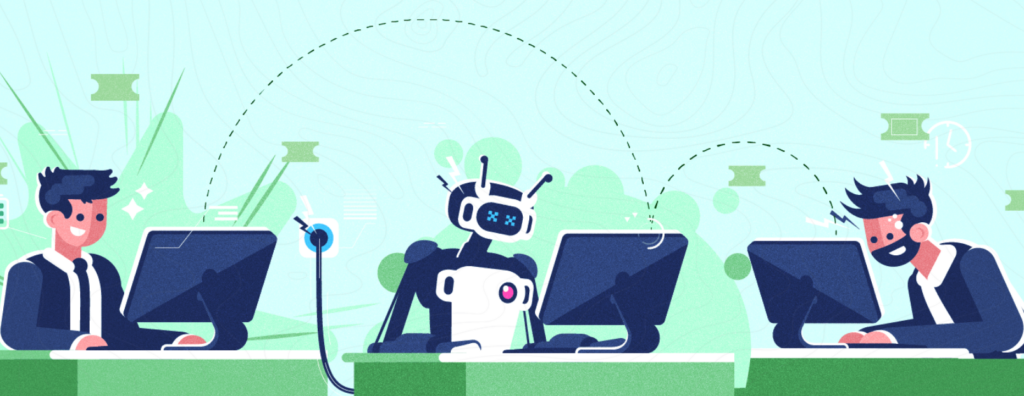
The big model represented by ChatGPT is now used in many enterprises for intelligent customer service applications. It can reduce the burden of human customer service by automatically answering common questions, solving simple problems and providing basic support. The Big Language model is trained by deep learning and large amounts of language data to understand and generate human language, enabling users to interact with it in a natural way. Such big models have achieved quite good results in answering some common customer queries and questions.
However, current big language models still have some challenges when applied in practice to intelligent customer service scenarios - it may generate wrong or inaccurate answers, especially for complex questions or domain-specific knowledge, which requires a higher level of intelligence. The future of online customer service departments will require not only more advanced algorithms and machine learning techniques, but also more precise natural language processing capabilities. This will put tremendous pressure on companies that are less technologically powerful.
In addition, as the number of users and visitors increases, intelligent customer service will need to handle mega concurrent requests in the future. This will require systems with special designs in a variety of areas, such as load balancing, high scalability, and high availability.
Many dialogue robots on the market now have a single fixed answer with relatively little variation, and there is still a gap with real human-to-human dialogue. Future intelligent customer service systems will need to further strengthen the adaptive and personalized services for user behavior. This requires the system to learn more user data and information, and adapt to different user behaviors to provide them with better services and experiences. How to improve user experience has become the main direction of intelligent customer service providers.
Specifically, improvements should be made in terms of humanized service, personalized service and anthropomorphic dialogue interaction.
First is humanized service. On the basis of accurate understanding of scenarios and intentions, attaching a warmer dialogue context can make the robot go further in anthropomorphism. Multimodal emotional computing is an effective way to achieve this step. At present, we are promoting virtual digital human customer service for human-computer interaction dialogues, in the process of combining emotional computing, which can recognize the emotional expressions conveyed by users through video, voice and text, so that intelligent customer service can respond with corresponding emotional feedback and create human-computer interaction with emotional understanding and temperature. This multimodal emotion computing technology is mainly implemented by two methods based on expert rules and machine learning. Among them, the machine learning-based approach automatically learns the classification criteria of emotional states by training models, which can better adapt to the expression of emotions in different domains and contexts, and the effect is relatively better.
The second is anthropomorphic dialogue-based interaction. Through scenario-based design optimization, such as question disassembly, topic inheritance, multi-round dialogue, contextual understanding, etc., the robot can bring a dialogue-based interaction mode that is closer to natural dialogue scenarios.
The third is personalized service. According to the customer portrait of a thousand people to provide personalized services, from multiple perspectives of semantic understanding, in addition to additional voice emotion discrimination.
The birth of the big model has undoubtedly injected a new "nutrient" into the field of intelligent customer service. This "innovation" is reflected in a number of aspects, including agent assistance and agent efficiency, small talk and pleasantries, suggestions for optimizing discourse, and the provision of corpus expansion.
Agent assistance and agent efficiency: In the past, intelligent assistance was more limited to single-round conversations, but the ability based on large models can quickly analyze and generate customer-oriented system support strategies, and such processing efficiency and results far exceed what can be achieved by relying on pure rules or pure knowledge base;
Small talk and pleasantries: a very key basic capability of intelligent customer service, which can help enterprises respond instantly to any incoming customer. In the past, the intelligent customer service gossip was mainly to pile up various non-business related corpus to the material database and reply to the content by retrieving the existing keywords from the database. Nowadays, it is possible to provide idle chats with the full help of large model capabilities to provide more decision-making basis and reference for agents and customer service in non-business areas;
Suggestions for conversation optimization: The conversation often determines the effect of customer service, and imprecise replies will directly lead to the loss of users. Through the powerful content generation capability of the large model, intelligent customer service can continuously iterate and optimize the conversation techniques to improve customer satisfaction;
Provide corpus expansion: In the cold start phase of intelligent customer service, it often needs enough corpus to enrich the knowledge base of similar questions to ensure a high enough solution rate and scene coverage of intelligent customer service at the early stage of launch. In the past, it was difficult for the corpus generation model to cover many vertical industries and fields. The large model has accumulated enough data and corpus in the general field, which can make up for the shortage of the corpus generation model and quickly generate similar questions to solve the problems of insufficient corpus and low scene coverage of intelligent customer service cold start.
Ultimately, the core of improving dialogue quality is to understand the scenarios of customers and users, and to be able to build a data framework for measuring gains and losses. With the combination of these two, there will be a cyclic feedback process to achieve good results through normal product iterations and be able to measure the ROI and share it with the actual business.
In the future, will AIGC completely replace customer service agents?
As the process of point wise of the whole society enters deeper water, more and more enterprises start to use digital tools - intelligent customer service system to do a good job in customer service, create the ultimate experience, and thus enhance the core competitiveness of enterprises.
Both vertical and general function customer service vendors hope to take enterprises to a higher level with the maturity of AIGC technology. However, there is still a long way to go to turn the dream into reality. For example, there is a need to measure the input-output ratio between cost and benefit, to ensure the privacy of user data and comply with relevant laws and regulations, and to ensure the usability and flexibility of the product in different industry scenarios.
As far as the customer service industry is concerned, the trend is to upgrade traditional manual customer service to intelligent customer service, but in the foreseeable future, intelligent customer service cannot completely replace manual customer service. They have different characteristics and positioning, and in service scenarios where complex issues are handled and high potential customers are followed up, human customer service is still indispensable. Therefore, companies need to consider the advantages of manual customer service and intelligent customer service according to their actual situation, so as to develop the best customer service strategy.
On the whole, AIGC has a broader development space in the future, and the AIGC and ChatGPT-like industries will develop at a high speed in the next two to three years, bringing further upgrades and changes to the Internet and enterprise service industries.
However, there are still many problems in terms of content quality, input cost, data security, privacy regulation and copyright ownership. In addition, better modeling methods are needed for long text generation, video generation, and incorporation of world knowledge; the training and reasoning costs of enhancing AI capabilities by increasing model parameters are high, and more economical and effective ways to improve performance need to be explored.